The aim of our research is to quantitatively describe the mechanisms of signaling and metabolic control mechanisms, which are molecular networks that regulate various biological phenomena, and to understand them as integrated “systems”. We are trying to understand cellular functions from the perspective of systems biology, using both experimental methods and computer simulations.
The essence of a signaling network is to encode information from a variety of inputs into a limited number of molecules. We are the first to discover a “temporal coding” in which the ERK pathway, AKT pathway, and insulin signaling regulate various physiological functions by encoding the input information into the temporal patterns of molecular activity. The aim of this laboratory is to clarify the mechanism of information processing in signaling networks from the viewpoint of the “temporal coding”. We use not only conventional molecular and cellular biological experiments but also various mathematical analysis methods such as simulations using differential equations, statistical models, and information theory. These methods are hypothesis-driven approaches in which hypotheses are formulated and tested based on previous knowledge. This is a common method in the life sciences so far.
In the life sciences, the word “information” has no definition and is used in a vague manner. On the other hand, in information theory, “information” can be defined as entropy. Our automated quantitative measurement technique, QIC (Quantitative Image Cytometry), allows us to measure how many bits of information a signaling network can carry. This is expected to reveal the characteristics of the signaling network as an information communication channel for the first time.
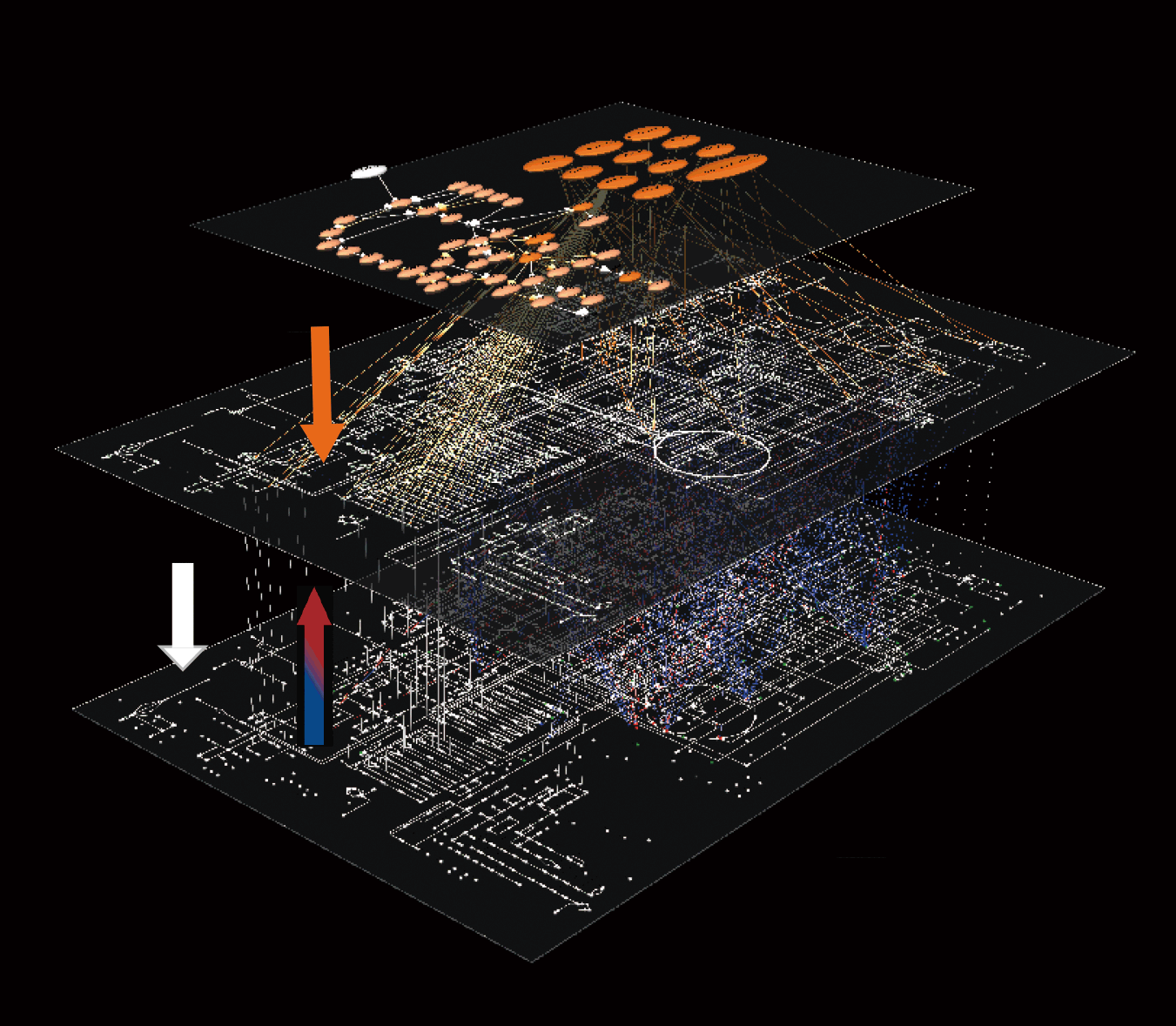
In previous analyses, the results of individual studies have been integrated to form a complete picture of the system (bottom-up approach). However, it has become apparent that this approach leads to various inconsistencies. The main reasons for this are the lack of comprehensiveness and multi-level hierarchy of the molecules measured in individual studies and the different experimental conditions. Therefore, it has become necessary to bring together technologies that comprehensively measure molecules at each layer (omics technologies), measure them under the same conditions, and integrate the data for analysis, i.e., trans-omics (top-down approach). It is impossible to perform these measurements in a single laboratory. Therefore, we have collaborated with phosphoproteomics (collaboration with Prof. Nakayama of Kyushu Univ. and Prof. Matsumoto of Niigata Univ.) and metabolomics (collaboration with Prof. Soga of Keio Univ.) to perform trans-omic measurements of insulin action under the same conditions, and to automatically analyze the global network of metabolic regulation by phosphoproteins from the omics data without manual intervention. We have established a method to automatically reconstruct the global network of metabolic regulation by phosphoproteins from the omics data in an unbiased manner without manual intervention. Trans-omics is a data-driven approach to unbiased analysis of large-scale data by computer according to a certain algorithm. This approach has the same concept as the recently developed big data analysis. One of the characteristics of our laboratory is that we conduct collaborative research with various laboratories, as each omics analysis is not something that can be done in a single laboratory.
Since the signal transduction and metabolic control mechanisms are composed of many elements and complex interactions, it is difficult to understand them only by using bottom-up methods that relate the information on individual molecules obtained from experimental observations to biological phenomena. On the other hand, a top-down approach, which extracts information from mere input/output data and estimates a model, cannot uniquely determine the model in many cases. In the future, we will shrink the unbiased global network identified in the top-down approach, identify the network motifs, analyze them individually, to elucidate the design principle of temporal coding. One of our next challenges is to combine bottom-up and top-down approaches in this way.
By integrating the above temporal coding with trans-omics, we are now analyzing individual-level data such as disease model mice and human trans-omics data, and we are now at the stage where we can publish our research results in the near future. The time has come for systems biology to make a direct contribution to medicine and drug discovery. I am looking forward to the future progress.
Our research methods are very diverse. In addition to conventional molecular cell biology experiments, we use various mathematical analyses such as simulations using differential equations, system identification, statistical models, and information theory. In order to perform these mathematical analyses, a large amount of highly accurate measurement data is required. Therefore, we are trying to introduce robots and automatic measurement methods to minimize the variation caused by human labor, and to achieve automation and high throughput.
1. Trans-omics of glucose metabolism
2. Temporal coding of insulin action
3. Information Transmission in Multicellular Tissues
4. Temporal patterns of human blood molecules in response to oral glucose ingestion
See also our movie of introduction of Kuroda Lab!
1. Trans-omics of glucose metabolism
The various phenotypes exhibited by living systems are regulated by multilevel molecular networks of metabolites, proteins, and mRNAs. Insulin-mediated regulation of metabolism is a good example of this, and it is known that signaling systems regulate glucose metabolism through phosphorylation of metabolic enzymes and transcriptional regulation. In order to elucidate these multilevel regulatory mechanisms, conventional biology has conducted multilevel analysis of only the periphery of some molecules or comprehensive analysis of only one level. However, these conventional bottom-up approaches are biased by the subjectivity of the researcher, and it is undeniable that key pathways and molecules may be missed.
To overcome the limitations of such bottom-up approaches, we have established the method of “trans-omic analysis,” a top-down approach that comprehensively measures multiple levels and reconstructs a large-scale network spanning multiple levels (Yugi et al., Cell Rep., 2014, Kawata et al, iScience, 2018, Yugi et al., Trends Biotech., 2016). Data-driven reconstruction of the multi-level regulatory pathways of insulin metabolism from “cellular big data” such as metabolome (in collaboration with Soga Lab., Keio Univ.) and phosphoproteome (in collaboration with Nakayama Lab., Kyushu Univ.) revealed that the regulation by insulin covers a wider network than previously thought. As a result, it became clear that insulin regulation covers a wider network than previously thought. Furthermore, the reconstructed insulin metabolic regulatory network was analyzed using mathematical models and molecular biology experiments, and a novel pathway that functions only in the liver was discovered.
We applied this trans-omics analysis method to mouse liver and revealed a large-scale metabolic regulatory network in the livers of healthy mice and obese model mice during glucose administration or fasting (Kokaji et al, Sci. Signal., 2020, Egami et al, iScience, 2021). We found that metabolism in healthy mice is rapidly regulated by gene expression via the Akt pathway and metabolites themselves, while in obese mice, much of the fast metabolic control is lost and slow metabolic control by gene expression is activated in glucose and lipid metabolism.
This methodology can be applied not only to insulin but also to the reconstruction of metabolic regulatory networks through signal transduction. It can also be used to reconstruct networks not only in cells and liver, but also in other organs and at the individual level. Taking advantage of this property, we will conduct trans-omics analysis of other multilevel regulatory mechanisms, organs, and individuals in the future.
[+] expand
2. Temporal coding of insulin action
Cells must use a limited number of signaling pathways to process a large amount of complex stimulus information about changes in the external world. To accomplish this, cells are known to use a combination of activating pathways to process information. In addition to this, we have proposed the concept of “temporal information coding,” in which information is embedded in the temporal patterns of molecules and processed by the cell.
[+] expand
Insulin regulates multiple responses in vivo, and its disruption is known to induce diabetes. It is also known that blood insulin shows multiple temporal patterns and the physiological significance of these temporal patterns has been reported, but the molecular mechanism by which these temporal patterns affect physiological effects has been unclear. Based on the concept of “temporal coding,” we hypothesized that multiple types of information are encoded in the temporal patterns of blood insulin, and that the insulin patterns individually regulates the response of target organs in a manner dependent on the encoded information. Therefore, we focused on liver cells, which are the first target organ of insulin and are most affected by the temporal patterns, and analyzed them using experiments and simulations. As a result, we found that the multiple information embedded in the insulin waveform is multiply encoded in the temporal pattern of AKT, which plays a central role in insulin action, and that the downstream molecules decode this information according to differences in network structure and kinetics. We found that the insulin waveform can regulate downstream molecules individually (Kubota et al., Mol. Cell, 2012). Such a mechanism is thought to be a general mechanism that exists not only in the insulin signaling pathway but also in other pathways. In the future, we will conduct experiments using animals to elucidate the mechanism of information processing by temporal patterns of insulin, with the aim of clarifying the existence of the concept of “temporal coding” and its mechanism in vivo.
3. Information Transmission in Multicellular Tissues
Cells adapt to their environment by regulating their responses to stimuli from the external environment, but due to the addition of noise to the signaling pathway, there is a possibility that the response cannot be accurately controlled. To evaluate how accurately a cell can respond in the presence of noise, it is necessary to perform information theoretic analysis to determine the variability of the response, and to obtain the value of mutual information using the following equation.
[+] expand
However, conventional studies have not been able to separate the variability of responses within the same cell from the variability of responses between cells, because the variability was determined by mixing the responses of different cells. As a result, not only intracellular variability but also intercellular variability has been treated as noise (Fig. 1A).
[+] expand
Recently, we succeeded in measuring intracellular and intercellular variability separately by applying repetitive stimuli to cultured mouse skeletal muscle cells C2C12 and isolated myofibers (Fig. 1B) (Wada et al., 2020). In addition, we investigated the tissue response, which is a more natural form of signal transduction for multicellular organisms (Fig. 1C). Interestingly, we found that the intercellular variability allowed the tissue to distinguish between more different stimulus intensities, which in contrast to previous studies in which the intercellular variability was noise (Fig. 2). We named this effect the “response diversity effect”. Furthermore, in vivo electromyographic measurements of facial muscles by electrical stimulation of the facial nerve showed that muscles in vivo responded more accurately than cells. We believe that the clarification of the precise response control mechanism due to the variability of the response of each cell will clarify the response control at the organ level, and will be useful in elucidating the mechanism of abnormal response control of organs due to diseases.
Fig. 2
[+] expand
4. Temporal patterns of human blood molecules in response to oral glucose ingestion
We are also conducting research focusing on the concentration of metabolites and hormones in human blood after glucose ingestion. After oral glucose or dietary ingestion, through absorption from the small intestine, blood glucose concentrations increase, and is stored and released in the liver and muscles, and these actions are promoted and inhibited by blood hormones. Insulin, the central blood glucose regulating hormone, not only inhibits the rise in blood glucose levels, is also involved in the control of many other metabolites, such as amino acids and lipids. However, the detailed temporal changes in the concentration (=temporal pattern) of comprehensive metabolites and hormones over long periods after oral glucose ingestion have yet to be examined. In healthy humans after glucose ingestion, therefore, we characterized the temporal patterns of comprehensive metabolite and hormones among individuals and among molecules by four features (Fig. 1, Fujita et al., under review, in collaboration with Soga Lab, Keio Univ.). (1) the decomposability into “amplitude” and “rate” components, (2) the similarity of temporal patterns among individuals, (3) the relation of molecules among individuals’ relation over time, and (4) the similarity of temporal patterns among molecules.
As a result, The amino acids whose temporal patterns were similar among individuals and whose relation among individuals was constant over time showed similar temporal patterns among the amino acids. The glucose metabolism-related molecules and free fatty acids, whose temporal patterns were similar among individuals but whose relation among individuals frequently changed over time did show similar temporal patterns among them. This result suggested that the metabolic control of amino acids was similarly conserved among individuals, but that of the glucose metabolism-related molecules and free fatty acids differed among individuals, although all individuals were healthy. The features of the temporal pattern of molecules after glucose ingestion that reflect differences in metabolic control among individuals will provide new clues for understanding physiology and pathology of systemic glucose metabolism between individuals and for personalized medicine in the future.
Fig. 1
[+] expand
On the other hand, we have also conducted research on a narrower range of target molecules, using mathematical models to describe the dynamic relationship between molecular concentrations after glucose ingestion. For example, in the study to describe blood glucose regulation by mathematical models using time-series data of blood molecule concentrations during and following oral glucose ingestion with various doses and durations, predicted that intermittent ingestion 30 min apart was the optimal glucose ingestion patterns that minimized the peak value of blood glucose level.
In addition, a study describing the feedback regulation of blood glucose and insulin by a mathematical model using time-series data of the blood molecule concentrations from a clinical trial in 111 subjects, including healthy normoglycemic and diabetic subjects with consecutive hyperglycemic clamp and hyperinsulinemic-euglycemic clamp conditions( Ohashi et al., 2015, 2018) showed, for the first time, that blood insulin removal is involved in the progression of type 2 diabetes. Thus, by using time-series data of molecular concentrations in blood obtained from human clinical trials and describing the regulatory relationships among molecules using mathematical models, we believe that we can devise appropriate health management methods and elucidate the degree of action (intensity of action) of molecules and the mechanisms of molecular action in the human body.